Fellow, affiliation:
07/2022-06/2024: Experimental Particle and Astroparticle Physics Group, University of Siegen
Exploring the multi-messenger Universe at ultra-high energies with a search for photons based on machine-learning techniques
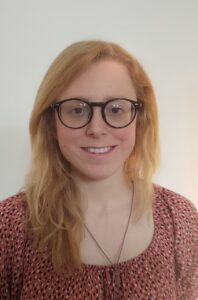
Dr. Eleonora Guido
Ultra-high-energy (UHE) cosmic rays, with energies above ∼1018 eV, have been recently proven to be mostly extragalactic, but there are still many uncertainties about their nature and origin, which is one of the most important open questions in physics. UHE photons are produced via the interactions UHE cosmic rays undergo both in their sources and during the propagation in the intergalactic medium, hence their observation is expected to be related to the composition and the energy distribution of the parent nuclei at the sources, to the processes occurring during their propagation and to the characteristics of the sources themselves. So far no UHE photons have been unambiguously observed, but
With this project I aim at developing a very promising method based on modern machine learning algorithms, which will significantly increase the capability to identify photons among the events recorded by the Pierre Auger Observatory, the most sensitive detector to UHE particles ever built.
It is a ground-based experiment covering an area of ~3000 km2 and observing the air-showers produced when a UHE primary particle enters the Earth’s atmosphere. A photon-induced air shower has a different particle content with respect to a hadron-induced one, which impacts on the shape of the signals recorded in the stations of the Surface Detector (SD) of the Observatory.
The most challenging part of this analysis is that it implies to deal with hundreds of variables. For this reason, I plan to exploit machine-learning techniques, which have been recently proven to be very well suited to deal with huge amount of data, like in this case. Besides, the Pierre Auger Observatory is also currently undergoing an upgrade which aims at better distinguishing the air-shower content, so the new upcoming data set is expected to further improve the accuracy of the discrimination method I proposed, which also appears particularly well-suited to this purpose since machine-learning techniques easily allow to include and combine data collected with different detectors.